Data aggregation is the process where raw data is gathered and summarized in a quantitative format, and is typically used to gain macro insights from large amounts of data. A commonly known example of data aggregation is the Consumer Price Index (CPI) from the Department of Labor which aggregates price changes in a wide variety of goods and services to track the fluctuation of the cost of living in the U.S. Unfortunately, despite the importance of data aggregation and its potential to improve decision-making, organizations still make major data aggregation mistakes.
The most common data aggregation mistakes
For businesses, data aggregation can provide insights into key metrics such as revenue growth, unit production, or earnings per customer. Internally, and especially with the improvements in analytics, data aggregation provides a steady stream of insight for teams of all sizes. As such, it’s become an essential tool across many verticals, such as finance, energy and utilities, and healthcare. Below, we’ll look at the most common data aggregation mistakes and how they can be fixed.
Insecure data sharing
Aggregated data is extremely valuable in a world with ever-increasing regulations on data sharing and security. That’s because aggregate data is anonymized and doesn’t carry the same restrictions or consent obligations as personal identifiable information (PII). This makes it easier to share, which can be vital in fields such as healthcare. However, a common data aggregation mistake is falling for the illusion of less risk, leading to oversharing and data breaches. To overcome this, data administrators need to have greater access control over all data sharing to ensure even aggregated data never leaves their control.
Duplicate data
Data duplication already contributes to unmanageable and costly data swamps, and it can also have major negative impacts on data aggregation processes. The double-counting of data can significantly skew results leading to false outputs and decision-making based on erroneous data. Data duplication occurs for a number of reasons, including problems during data integration and lack of metadata usage. Avoiding the impacts of duplicate data on data aggregation is an ongoing governance process that can be assisted through the deployment of custom data architecture.
Poor process methodology
Data can only be as useful as the questions asked of it. This becomes apparent through poor query formation leading to discrepancies between what decision-makers think they’re seeing and what the collected data actually says. For example, a “running daily average” of energy consumption per customer would vary significantly depending on whether it was a weekly, monthly, or quarterly dataset. For effective data aggregation results, data scientists need to be consistent and clear about queries and metrics. This way, outputs such as % change are always from a relevant comparison.
Incomplete data
To extract data from multiple sources and curate datasets that deliver useful, actionable information to downstream users is a key role of data operations. This includes summary insight derived from the aggregation of multiple data points. However, gathering the required data for querying may be difficult or require lengthy ETL processes, leading to incomplete datasets. With data aggregation taking such a broad view in its outputs, this incomplete data may not be initially noticed but nevertheless has an impact on downstream usage and decisions made.
Data moving at different speeds
Even with access to cleaned and complete datasets, data aggregation mistakes can still occur. One of the biggest reasons for this is the speed of data flows, which can vary significantly depending on storage, access to siloed data, and how data entering data lakes is processed. This can create issues for data aggregation points, especially those being used in real-time dashboards. For example, imagine a network of IoT sensors measuring transformer throughput which then informs a centralized dashboard used throughout the utility. If one portion of the network is even 30 minutes behind, the aggregate data will constantly be off.
How to solve data aggregation mistakes
Data aggregation can be a very useful tool for DataOps and provides usable and interesting insight for the rest of the organization. However, overcoming data aggregation mistakes means also overcoming significant challenges in terms of data consistency, avoiding unnecessary migrations which lead to duplication, and giving admins greater control over how data is used and how datasets are created for analysis.
Deploying a virtualized data platform can solve or reduce the impact of many of the mentioned data aggregation mistakes. By creating an interoperable virtual layer between data storage and processing, data will always be available for query without the need for time-consuming data migrations. Plus, since data analysis and sharing takes place in secure execution environments, the chance of data leakages during these processes is minimized.
Data admins are also given much greater access control, down to a granular row and column level, which is especially important for ensuring compliant data sharing, even of aggregated data. This greater control also allows for more precise and consistent querying and dataset creation.
Intertrust’s data virtualization solution, Intertrust Platform, is already helping organizations streamline data operations, improve ROI, and ensure compliance. To find out how Intertrust Platform can help you achieve your data operations goals and overcome data aggregation mistakes, you can learn more here or talk to our team.
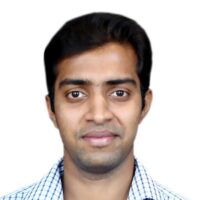
About Abhishek Prabhakar
Abhishek Prabhakar is a Senior Manager ( Marketing Strategy and Product Planning ) at Intertrust Technologies Corporation, and is primarily involved in the global product marketing and planning function for The Intertrust Platform. He has extensive experience in the field of new age enterprise transformation technologies and is actively involved in market research and strategic partnerships in the field.